1. Analyzing Online Reviews - Patterns of Research, Methodological Issues and Empirical Results
Reinhold Decker, Bielefeld University
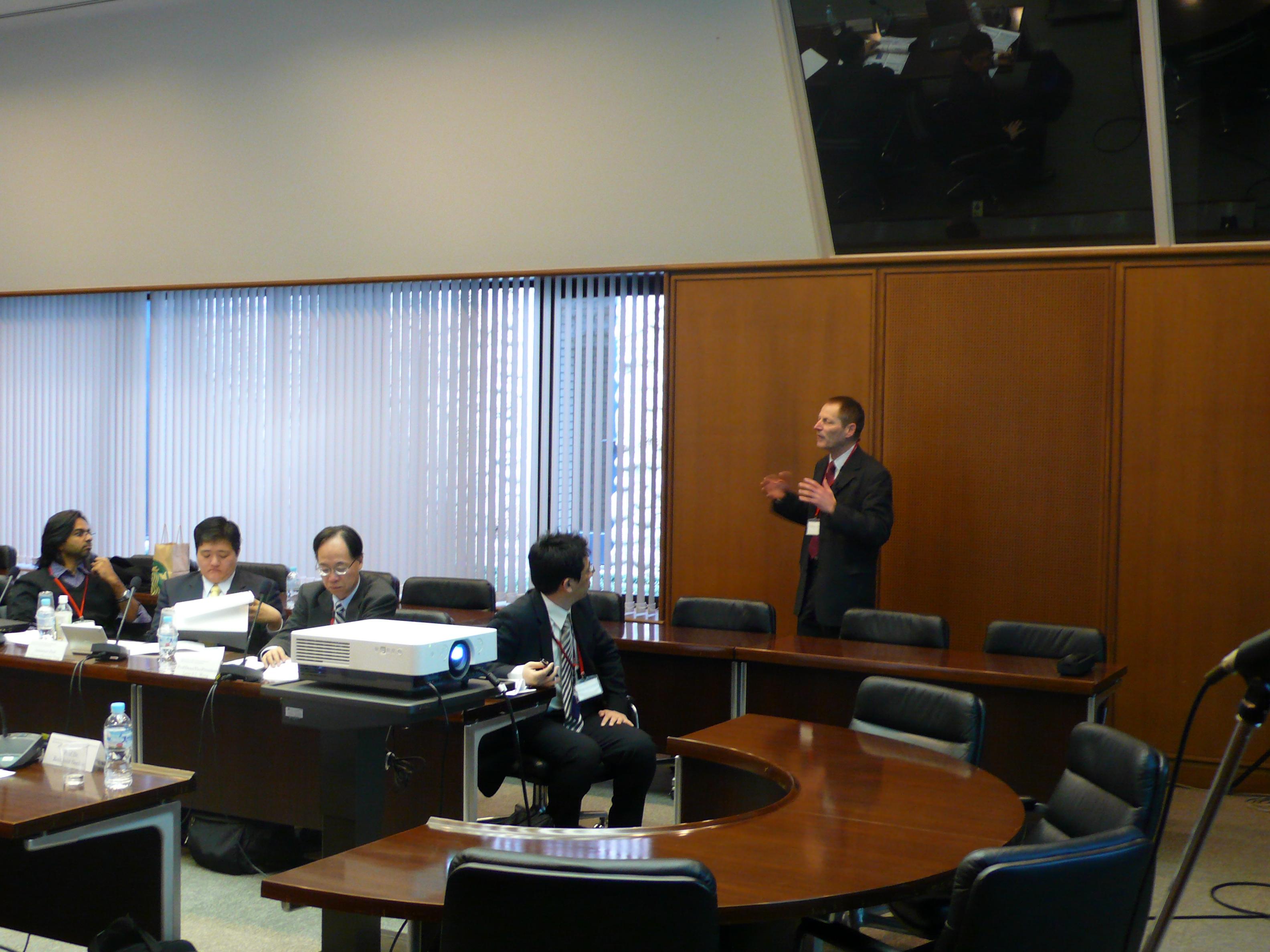
The quantitative analysis of large amounts of online reviews has attracted increasing attention in data mining and marketing research in the recent past. Typically, consumer reviews, as posted on websites like amazon.com, epinions.com, or airlinequality.com, consist of different components which are assumed to be interrelated, particularly “star” ratings, pros and cons, as well as free format texts. Mining and analyzing such data may help to better understand consumers’ opinions and preferences.
Against this background the talk deals with different methodological issues that make the profound analysis of review data an exciting but also challenging undertaking. Based on several datasets representing both products and services, we present current findings on the plausibility and consistency of online reviews as well as the inevitable uncertainty and partial incompleteness of evaluations. Furthermore, aspects with regard to content, particularly heterogeneity and distinctiveness, as well as the cultural dependency of evaluation patterns are discussed. Most of the presented findings result from the specific application of econometric and stochastic methods or models.
2. Using Image Analysis for Improving Marketing Strategies - Algorithms for Image Feature Extraction and Model Building
Daniel Baier, Brandenburg University of technology Cottbus
Nowadays, the diffusion of smartphones, tablet computers, and other multipurpose equipment with high-speed Internet access makes new data types available for data analysis and classification in marketing. So, e.g., it is now possible to collect images/snaps, music, or videos instead of ratings. With appropriate algorithms and software at hand, a marketing researcher could simply group or classify respondents according to the content of uploaded images/snaps, music, or videos. However, appropriate algorithms and software are sparsely known in marketing research up to now. The paper tries to close this gap. Algorithms and software from computer science are presented, adapted and applied to data analysis and classification in marketing. The new SPSS-like software package IMADAC is introduced.
3. How to Grow Your Network On Facebook: Drivers of Number of Friends
Dirk Van den Poel, Ghent University
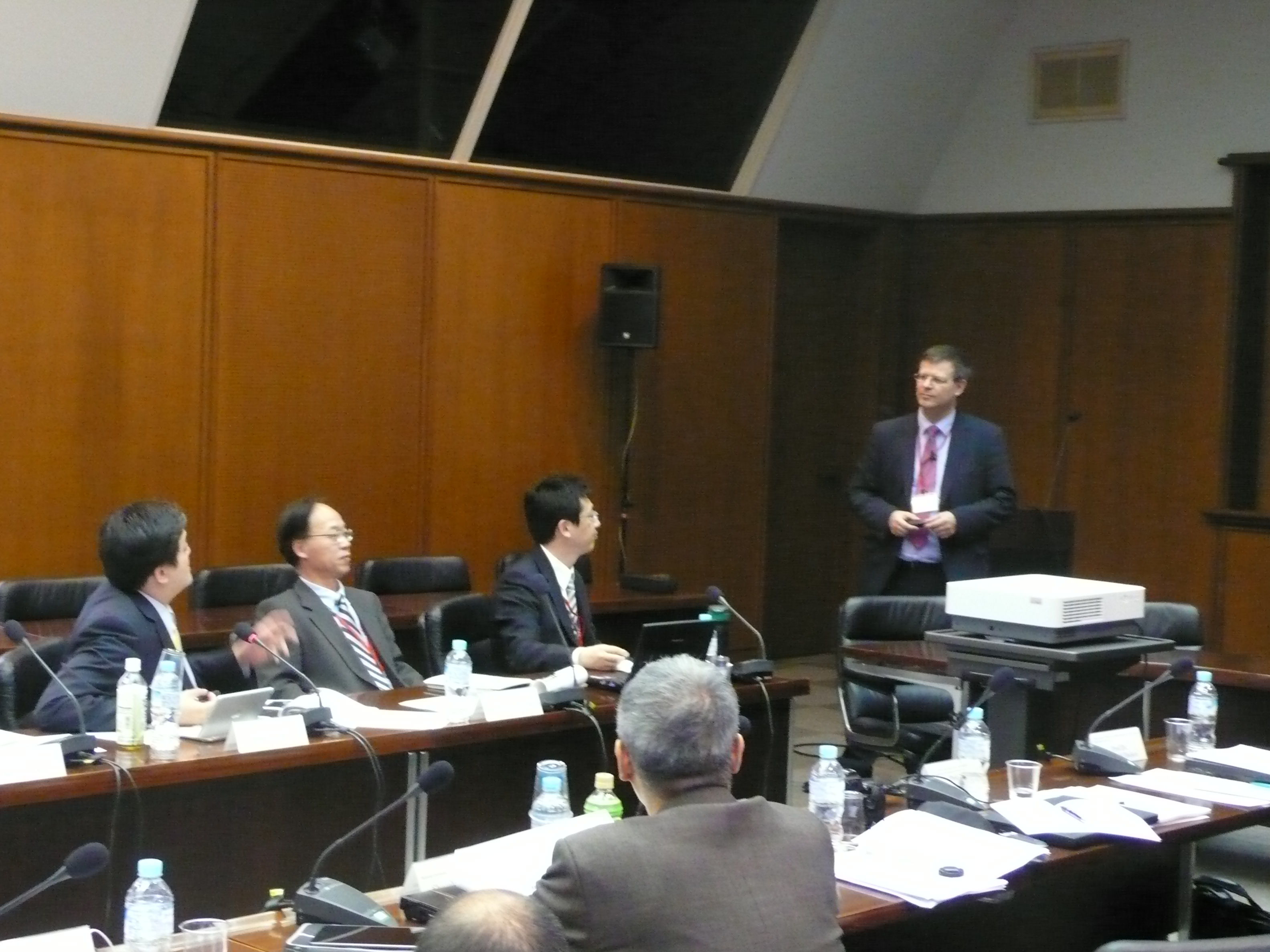
This study investigates the advantage of social network mining in a customer retention context. A company that is able to identify likely churners in an early stage can take appropriate steps to prevent these potential churners from actually churning and subsequently increase profit. Academics and practitioners are constantly trying to optimize their predictive-analytics models by searching for better predictors. The aim of this study is to investigate if, in addition to the conventional sets of variables (socio-demographics, purchase history, etc.), kinship network based variables improve the predictive power of customer retention models. Results show that the predictive power of the churn model can indeed be improved by adding the social network (SNA-) based variables. Including network structure measures (i.e. degree, betweenness centrality and density) increase predictive accuracy, but contextual network based variables turn out to have the highest impact on discriminating churners from non-churners. For the majority of the latter type of network variables, the importance in the model is even higher than the individual level counterpart variable.
4. Recent Studies in Social Networks and Privacy Preservation
Leon S.L. Wang, National University of Kaohsiung
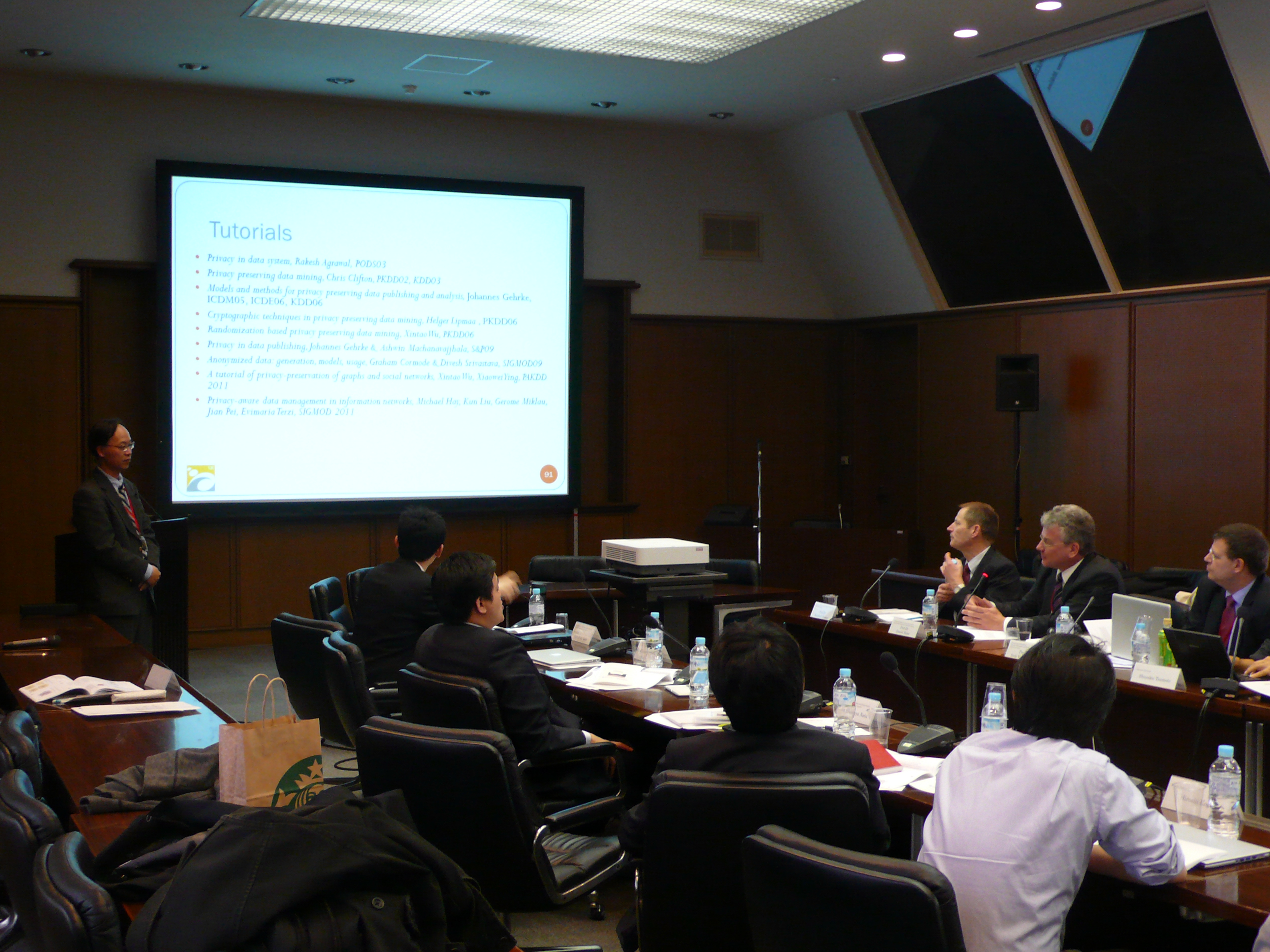
In recent years, social network research has advanced significantly. People perceive the Web increasingly as a social medium that fosters interaction among people, sharing of experiences and knowledge, group activities, community formation and evolution. This has led to a rising prominence of social network analysis and mining in academia, politics, homeland security and business. However, growing popularity of on-line social networking, not only brings the convenience of information sharing but also concerns of privacy breaches, as sensitive information, through links and inferences, individual's identity, health, financial status, political affiliations, etc, might be disclosed. In order to preserve privacy of users, anonymization is required prior to attempts to make the data more widely available to public. In this talk, we will first review the basic concept of social networks analysis and mining, and then concentrate on introducing recent studies in privacyy preservation in data publishing, data mining, networking publishing, location publishing, and mobile publishing.
5. Identifying Key Users from Social Network Data
I-Hsien (Derrik) Ting, National University of Kaohsiung
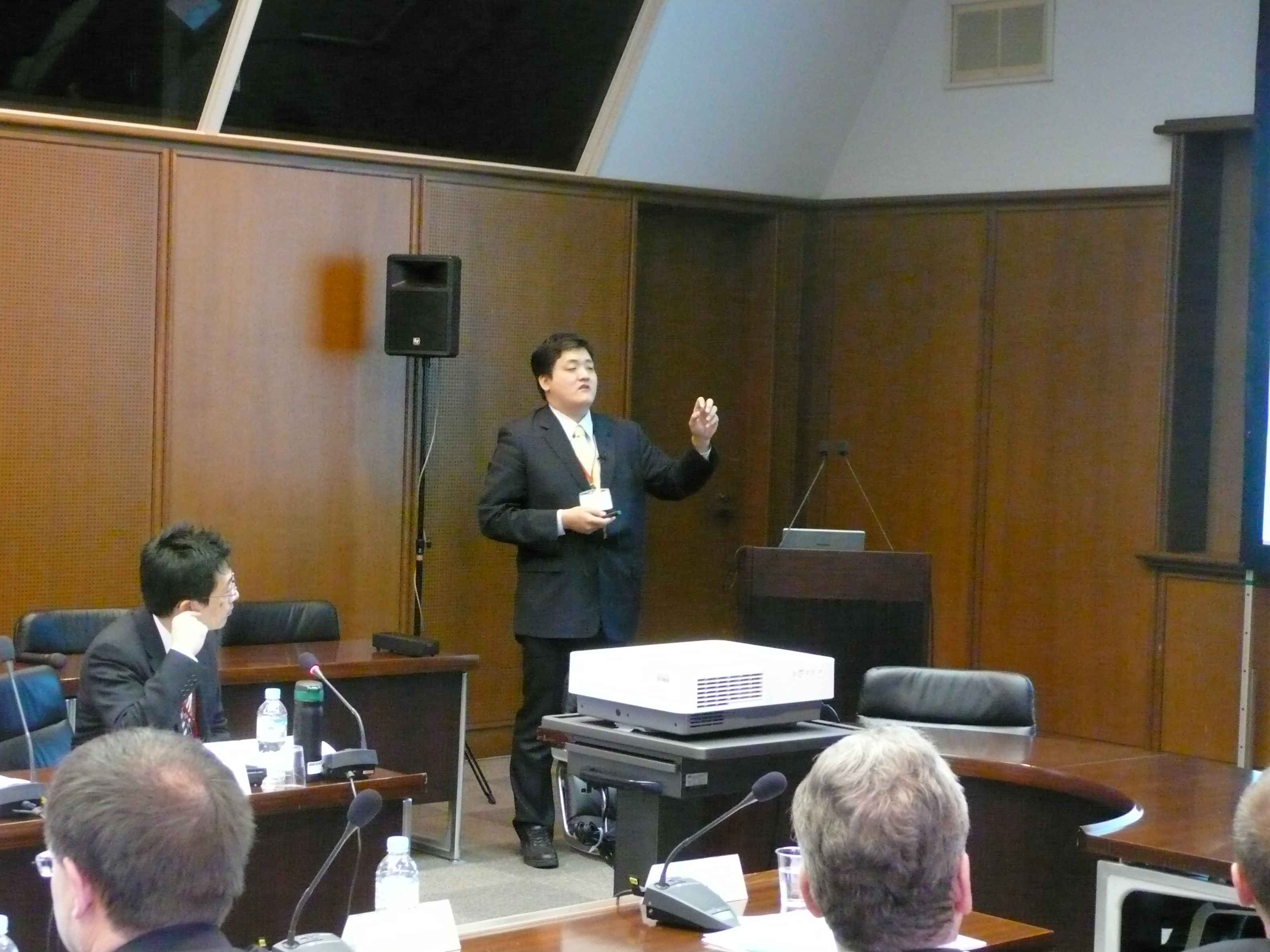
In recent years, social networks has becoming a very popular topics in the area of information technology. Large amount of social data have been generated due to the rapid development of web 2.0 and social networking website. In order to apply the social data, social networks analysis is now a very important methodology to analyze social data, especially to identify key users. The identification of key users is essential for many application, because it can help to get useful information or to transfer information in social networks more efficient. In this talk, I will share the research results of identifying key users from two different types of social networking website, which are Microblogs (Plurk.com) and Virtual Community (IMDb). The approaches about how to identify key users from the two social networking websites will be introduced as well as the evaluation of the quality of the identified key users. In addition, potential applications about key users in Business will also be discussed as the conclusion of this talk.
6. Knowledge-centric Solutions for Performance Management
Maitreya Natu, TaTa Consultancy Services
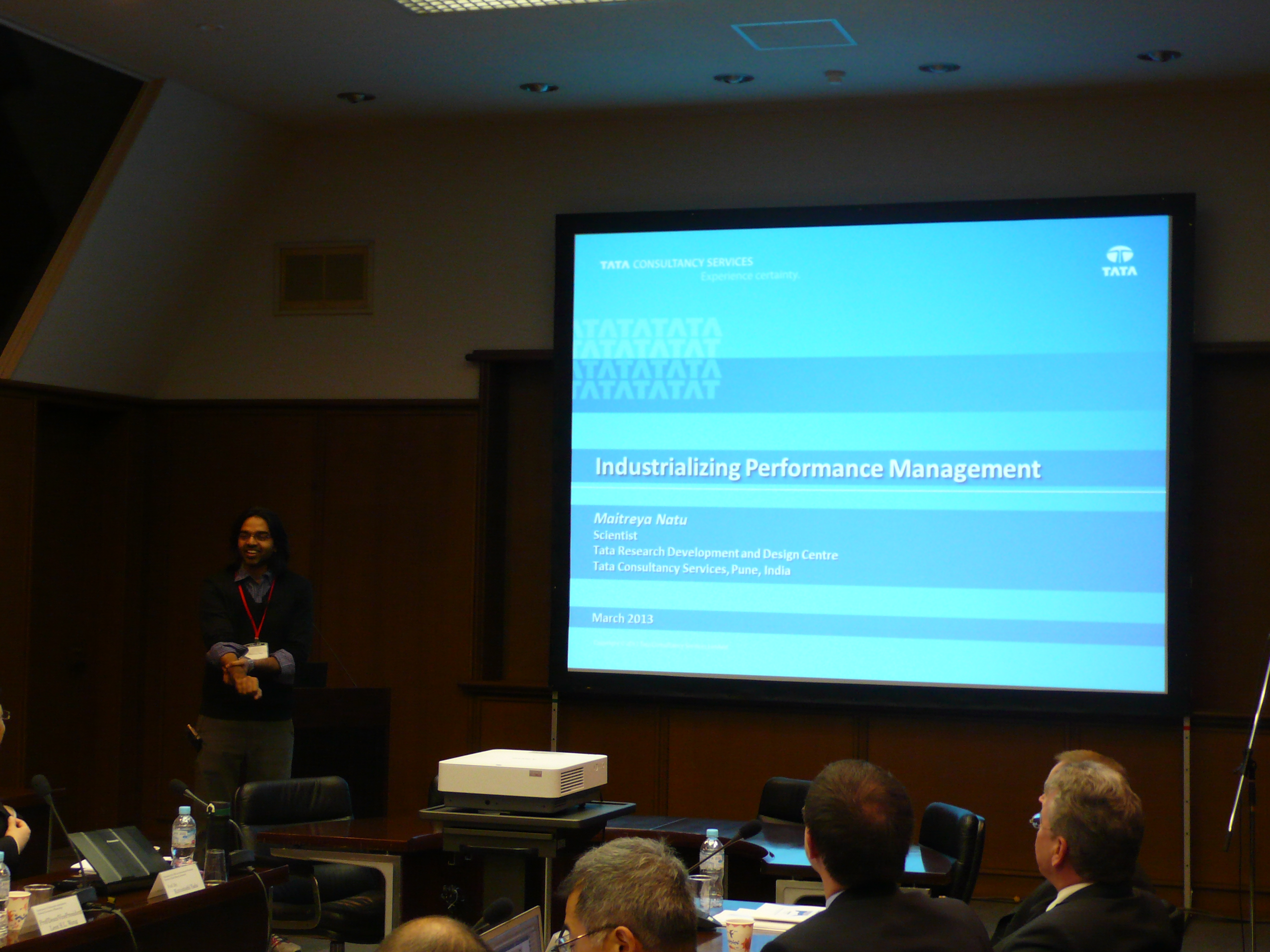
With the increase in size and complexity of enterprise IT environments, manual approaches to performance analysis and management are fast becoming inadequate. Automation is essential for managing complexity. Over the past several years, many statistical and machine learning techniques for automated performance analysis have been developed. These techniques have proved to be very successful in identifying interesting observations (e.g., performance events and trends) automatically. To achieve the holy-grail of "automated performance management", however, one needs to go beyond observations to automated derivation of insights and actionable recommendations. In this talk, we will argue that this requires formalization and codification of tacit knowledge about the system, its components and the domain of performance engineering. We will discuss some initial ideas and challenges in developing such knowledge-centric solutions for automated performance management and illustrate the concepts through an example.
.